Heads up: Long read.
In 1712, Thomas Newcomen invented the first steam engine.
The noisy, sputtering, fire-belching machine rocked a piston and chain back and forth to pump water out of mines.
When the piston reached the bottom of the housing1, it wheezed a sooty mix of steam and smoke before traveling back to the top.
The first example of this engine replaced a team of 500 horses!
The new system was a great advance over using horses for mine pumps.
Outside of the mine pump industry, this new engine was nothing but an interesting novelty. The new system was loud, slow, and enormous.
Factories that had used water wheels for power before this invention didn’t even consider changing over to a steam system.
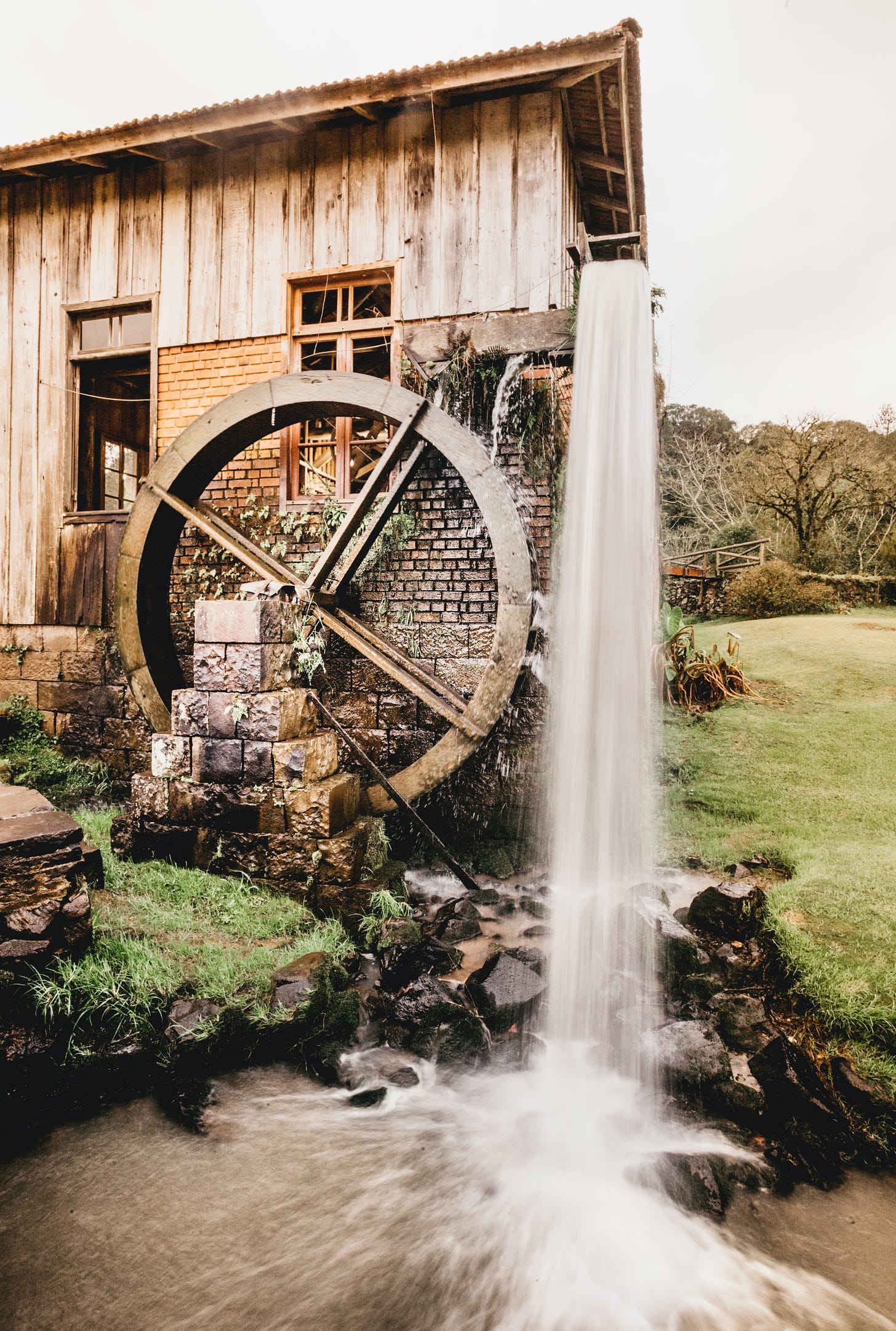
Decades later, James Watt was working as an instrument maker and at the University of Glasgow.
As a personal favor to a friend, Watt used his skills as an instrument maker to repair a broken Newcomen engine.
He made the machine function exactly as it was initially designed.
He wasn’t happy with that.
Over the next new years, he experimented with various designs and improvements to the system.
The eventual result was the groundbreaking Watt engine.
Twice as fuel efficient, many times faster, and many times smaller, the Watt engine was immediately adopted by factories.
Far from being bound to niche industrial applications, this engine had the potential to—and did—power the entire Western world for nearly two centuries.
Watt had freed humanity from the confines of nature and unleashed the power of steam onto the planet.
Improvement wins inches while innovation wins wars.
Why I’m writing this
AI search interest has spiked since the release of Chat GPT2.
Even people outside of the technology industry are often talking about Chat GPT—what it can do, what it can’t, if we should be afraid of it, etc.
Everyone seems to have some prediction about how a new AI tool will disrupt {Insert industry here} forever.
For the past year, the AI mania has run unabated.
If you could even mention AI on a quarterly investor relations call, your stock went up.
But eventually, all that goes up must come down.
The AI bubble looks like it’s beginning to pop.
It’s an unfortunate time for speculators financially tied to realization of widespread AI success.
but a perfect time to soberly consider what the real short- and mid-term future of MarTech looks like with new AI tools and practices.
In this previous article, I linked a non-hype list of ML/AI resources. They’re continuously updated on the main GitHub page—check it out if you’re curious about what’s the real current state of the union on the technology.
Demystifying Marketing Automation
Check out this article to get a simplified primer of what marketing could look like in a highly automated future, how to prioritize initiatives, and what is already obvious as hype.
What Does Disruptive Innovation Usually Look Like
Disruptive innovation takes what the rich had in the past and gives it to everyone
a personal translator ($160/hr) became Google Translate (~$0.0001/word) [2023 USD]
a traditional American vehicle (~$2750) became a mass-produced 60’s Toyota (~$1700) [1960s USD]
a vacuum tube radio (~$800) became affordable transistor radios (~$160) [2023s USD]
Innovation follows a few common trends.
Surprising that innovation has patterns, right?
I’ve previously written about disruptive innovation in these two articles linked below.
The Power of Disruptive Innovation: Part 1 (ID-ing Innovation Patterns)
The Power of Disruptive Innovation: Part 2 (Guarding Against Disruption)
In summary, disruptive innovations usually start in a barely profitable industry.
The low profitability provides protection to shelter the innovator from the raging storm of competition, and the protection provides a path to the profitability.
It’s hard to disrupt big companies.
It’s easier to take many small bites.
Evaluation Areas
In this post, I’ll give a short summary of what changes are likely to come down in each of the following areas. Follow-up posts will go more in depth for the relevant sections.
The color of emoji shows how likely a specific area is for generative AI-based disruption within the next 5 years. 🟢 is likely, 🟡 is a toss up, 🔴 is unlikely
🟢 AdTech
🟢 Social Media Marketing
🟢 Content Marketing
🟢 Brand Marketing
🟡 Customer Experience
🟡 B2C Pricing
🟡 Marketing Pipeline Management
🔴 Traditional Marketing
🔴 Account Based Marketing
🔴 B2B Pricing
🟢: Very likely to change significantly. The skills required to succeed will be different from 2019
🟢 AdTech
🟢 Social Media Marketing
🟢 Content Marketing
🟢 Brand Marketing
Especially for B2C brands, these 4 areas will likely require understanding and experience with AI tools within the next few years.
Customers bandwagon, customers accelerate pump-and-dump cycles, customers get REALLY into fidget spinners before forgetting they exist.
For companies that want to be on the right side of consumer sentiment, being able to react quickly is paramount.
AI tools will dramatically change the landscape of customer-facing marketing because they are so fast to react.
If a national news story goes viral about a cute dog saving a child, then companies that generate assets that include similar-looking dogs quickly will win hearts of their audience.
If TikDock—a new boating-themed social media—has a strong impression on a company’s audience, an AI-managed media mix tool could quickly snap up undervalued ad spots for thier products before their competition
New customer-facing content can be generated with up-to-date materials, and previous posts can be kept up to date with current offerings, pricing, and information if an AI content manager is left in charge
These areas of marketing are ripe for AI advancements, and will be the first to be disrupted in the mix.
🟡: Moderately likely to change. New skills will be expected for many industry positions, but may not be required for all.
🟡 Customer Experience (CX)
🟡 B2C Pricing
🟡 Marketing Pipeline Management
These areas, while still generally customer-facing, require more forethought and backend management than the category above. The systems that will disrupt these areas of marketing will likely be hybrids of humans and AI tools.
For CX a good, human understanding of what your customer journey is still vital to being able to design good experiences. AI tools will be able to dynamically identify Moments of Truth within segments—far too much multivariate analysis for most existing CX departments.
B2C pricing has already changed significantly since the days of paper labels on items. Increasing dynamism in international trade, heightening global inflation, and increasing customer understanding of surge pricing models will make dynamic AI Willingness-To-Pay pricing models a requirement for modern B2C companies to maximize profit.
Marketing pipelines are still plagued with delays from approvals, edits, confusing versioning, etc. The problems of yesteryear have yet to be solved by a slick tech tool. A context-aware AI tool will be able to re-prompt users to keep marketing pipeline projects on track with necessary approvals on the right versions.
🔴: Unlikely to change. New tools may exist, but the core skills and competencies required will be similar to 2019.
🔴 Traditional Marketing
🔴 Account Based Marketing
🔴 B2B Pricing
These areas will likely involve increased capabilities of existing tools (e.g. a sentiment analysis tool on calls attached to a CRM for tracking prospect moods), but are human-driven and slow to adapt.
These areas have changed less than other marketing areas in the last 15 years and are likely to retain that inertia.
Traditional marketing will still require human touch. The genius 2014 Budweiser Super Bowl ad was a departure from ongoing trends, so an AI tool would recommend against it. It’s also the most remembered of any Super Bowl ad slot in the last decade
Account Based Marketing. People are emotional buyers. Knowing how to get people en masse to purchase an item is a data-driven exercise discussed above in B2C. Selling at an individual level will still require human touch and is unlikely to be disrupted by AI in the near future
B2B Pricing. See above^. Small markets do not do well with large data models.
Conclusion
The AI bubble may be popping, but the real results from the new technology haven’t yet been felt.
I’m hoping my forecasts for these items are sober and realistic enough to keep this article from aging like this Krugman quote:
Do you agree or disagree? Send me an email or add a comment if there’s an area that I left addressed or misrepresented.
Ironically called Top Dead Center in engine dynamics
And all of the other Generative Pretrained Transformers (GPT)s that have come in it’s wake