Analytics? I thought this was MarTech?
Yes, I know the introduction article talked about a soft entry into Marketing Technologies and getting a clearer picture of that world.
Q: So why am I starting with Analytics?
A: MarTech tools and practices change extremely quickly, but the underlying ideas don’t.
I’ll be maintaining this newsletter as a way of keeping you as up-do-date as possible, but there will be some areas that I won’t be able to keep up with. This first series will always remain true, and the framework laid out here will be useful for managing and evaluating the claims and goals of future Marketing Technologies
If you ever need to figure out how to judge the claims and merits of something new that I haven’t covered, use the info in this series to guide your evaluation.
Good luck!
Jumping In
If you’re a marketer or marketing analyst, you’ve certainly heard hype about “Self‑Serve marketing tools” or “democratized data”.
These are tools that claim to give marketers the ability to reign in Big Data and design effective marketing interactions for customers without waiting on shared data resources & IT to produce analysis for them.
They mean that you don’t have to wait for someone ‘techy’ to do work for you—you can give it a go today.
If you’re not sure how to start, keep reading.
Series Structure
This is a 6-part series that will:
walk you through the zero-to-hero analytic planning framework
show how to generate a business-relevant hypothesis
showcase with real math and code how analytics are done
explain common errors to avoid
give examples of how to show results to peers or managers
Why Should I Care?
Self-service tools open an extremely powerful opportunity for marketers to better understand their customers and the highest levels of marketing officers have already noticed.
According to Deloitte’s recent Building the Intelligent Creative Engine report:
[Deloitte] surveyed 556 global …(CMOs) and asked them to identify the top skills of their highest performers. Analytical expertise edged out creative skills in almost every industry…something that would be almost unheard of 10 years ago
The biggest stumbling block that marketers run into while trying to flex their analytic skills is that currently available tools are designed for someone with a data science background—a situation at odds with the reality of most marketers’ experience.
For marketers who have previously feared diving into the data, fear no more!
In this article series, we lay out the framework for approaching a new marketing data problem, discuss some potential pitfalls to avoid, and show how to walk through the process on a sample direct marketing data set.
Framework Overview
A woodsman was once asked, “What would you do if you had just five minutes to chop down a tree?” He answered, “I would spend the first two and a half minutes sharpening my axe.”
—Anonymous
Imagine exploring an unknown mountain range to find a buried treasure. You know that the treasure is buried below trees that sit in the shape of a heart and the general location, but not much else about the environment or how get there.
There are swamps and areas full of dangerous animals, but you’re confident you can get to the treasure.
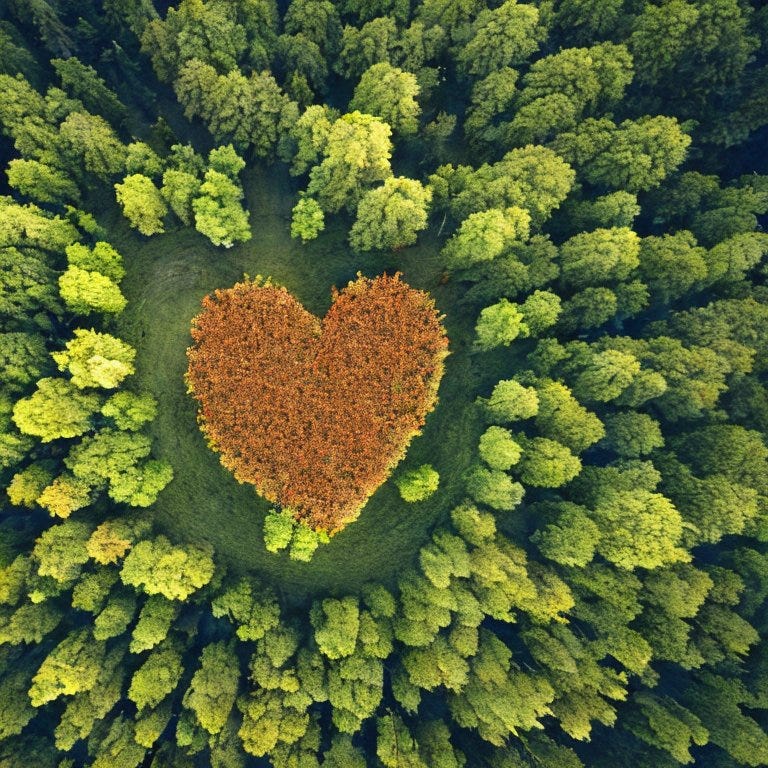
Exploring a new marketing dataset is not too far off (except there are generally fewer wolves in a modern office). Finding a useful relationship in your data can be buried treasure, there are pitfalls that can slow you down, and you often know general area for finding those relationships in advance.
Whether you’re searching for buried treasure or trying your hand at marketing-focused data analysis, following the 5-step process below to guide your efforts will allow you to better serve your customers in the modern world of data
1. Orient: Design A Real-World End Goal for Analysis
Make sure you don’t miss the forest for the trees by figuring out which way is north before beginning any analysis
Define what a successful business result would be for your analysis and align your effort to that goal
Your efforts should result in a business hypothesis that is testable, falsifiable, and would meaningfully affect business performance
2. Observe: Run Descriptive Statistics on Relevant Data
Build the edges of the map around your true north by getting to know your data with descriptive summary statistics
Calculate relevant descriptive statistics for relevant data before diving into analysis so that you familiarize yourself with the shape and range of the data
Understanding the bumps and edges of any dataset through descriptive statistics helps inform future analysis
3. Avoid: Consider Measurement Artifacts and Outliers
Plot out potential sinkholes and traps by considering measurement artifacts and outlier data
Understand how your data points were collected and how the measurements might affect the results
Create a plan to define and deal with outliers before doing your analysis
4. Act: Analyze Relevant Data
Once the first draft of the data landscape is mapped out, start exploring your data through appropriate mathematical tools
Continuously ensure your efforts are aligned to the goal you set in Step 1
5. Verify: Validate Your Conclusions
After you’ve done your exploration and analysis, set up ways to continue ingesting and testing new information to ensure you arrived in the correct location
Use future business data to validate your analysis by testing for spurious correlations and mis-assigned causation with future data
If you know anyone who could benefit from learning how to manage their own analytics, feel free to send them a link to the article.
This post will always remain public and free
If you want to stay in the loop for future parts, make sure to subscribe to the newsletter.