Confronting the Complexity Crisis in SaaS Data Analytics Tools
How AI Promises to Liberate Users from the Clutches of Overly Complex SaaS Tools
🔥Hot Take🔥: SaaS data analytics tools are all about the same.
Granted, I’m not quite same level of 'anti-SaaS’ as this guy…
…but I still think there’s a lot of product-feature echo chamber within SaaS products.
Yes, there may be better UI or proprietary model XYZ.
But how much of that would your customers know about if the marketing materials didn’t tell them that it mattered?
Data analytics tools today are so complicated, that many avid users STILL aren’t able to keep up with all their features and integrations.
The Path to Better Data Tools
Not limited to the customer-facing use cases we saw in last weeks post, data analytics can be greatly improved by AI as well.
How AI Can Create Interactive Content On The Fly
Last week’s post discussed the advantages that interactive content has over traditional, passive content—like reading or viewing. After listing all the benefits of interactive content (engagement, better data, customer retention), it seems like a done deal.
There are a few major ways that new AI advancements can improve data analytics tools in the near future:
Natural Language
Users will be able to ask a tool to do something without the highly technical knowledge required to script it
Better Suggestions
Improved Feature Prioritization
Natural Language Tool Usage
Let’s pretend you’re going to create a non-linear regression to determine if you’ve saturated an audience with advertising on one channel. You want to pull data from your Data Warehouse and see where on a logistic curve you fall.
You have to navigate through menus within menus:
Models>Regression>Nonlinear>Logistic>Import>DataWarehouse…
Nearly 37% of internet users abandon form tasks where their effort expectations and reality don’t align.
In an effort to cram more functionality into existing tools, developers for data analytics platforms have created their own ‘dark patterns’ of menu mazes.
A user who already understands the math and where the data is coming from would be upset at how difficult making this (somewhat) simple model is.
Scott Brinker—of Cheif Martec—is thrilled about the future of SaaS tools.
He sees AI as the turning point where a user can ask a tool for an outcome and it could do the processing in the back end.
He calls it “The amazing turning point when martech systems complexity and martech UX complexity diverge”
And his future estimation is no less valid for data analytics tools. Instead of needing to know to go through the menu labyrinth like you currently do.
Models>Regression>Nonlinear>Logistic>Import>DataWarehouse…
😓
You could ask a tools AI chat “Can you create a logistic model for cohort conversion rates based on the historical marketing data from our data warehouse?” and it could create it for you from there.
🥳
Hightouch just released a system that lets you ask natural language questions to query your data earlier this month—it’s already happening.
This natural language functionality unlocks the hidden value from existing tools—creating meaningful customer experience differences from the features that developers worked hard to create, but are currently hidden from or barely used by most users.
Improved Suggested Analytics
Gone are the days of manual sifting through datasets to uncover insights.
The future lies in AI's ability to not only understand our queries, regardless of how they're phrased but to also suggest the best analytical processes for our needs.
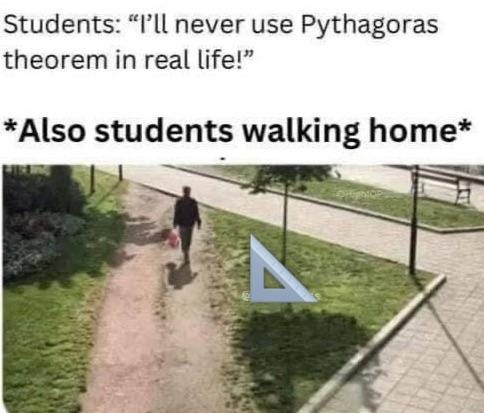
Imagine initiating a data analysis project and having AI suggest a multi-faceted approach—based on the data you fed it—that includes predictive modeling, sentiment analysis, and trend forecasting, all tailored to your specific data set and objectives.
This capability would significantly lower the barrier to entry for complex data analysis, enabling users from various backgrounds to leverage data in decision-making—true data democratization.
It would ensure that users don't miss out on valuable insights because they didn't know which analysis process to use.
For instance, when exploring customer feedback data, AI could suggest a sentiment analysis to gauge overall customer satisfaction, followed by a churn prediction model to identify at-risk customers, providing a comprehensive view of customer sentiment and behavior.
Without industry expertise and a good idea of where you’re going, that kind of analysis would be slow and difficult. With newer AI tools, an up-front list of all data fields needed would be generated, and IT could help you all in one go.
Improved Feature Prioritization
Margaret-Anne Heyland, a CX expert, often recommends that her clients begin their customer experience journey in the front lines. That usually means listening to a customer service rep resolve a complaint.
Note: Margaret-Anne is publishing a full article about the importance of the call center in the next few weeks. Keep tabs on the evolv blog page to see when it comes out.
By hearing the times when customers are highly emotional—either positively or negatively—and what terminology they use, you can design a more customer-centric journey. She will begin projects by sitting with the call center reps, hearing calls in real time, and seeing what tools / information the reps have available to solve problems.
Executives who listen to the literal voice of their customer are able to better understand their needs. But this process is often anecdotal and time-consuming.
AI can improve VOC by aggregating and analyzing the calls ‘recorded for quality assurance’ and highlighting the most common problems. They can also aggregate cross-channel information.
For example, if people email about a certain issue because they want to include screenshots, then a leader who sits only in the call center might miss the email issue.
By casting a wider net across all customer service interactions, AI can help prioritize feature addition.
For more info on how looking across channels instead of in segmented groups changes things, check out my previous post on the topic
Imagine 50 customers complained that they couldn’t export their data—but if the complaints were spread out across calls, emails, and the “Suggestion” form submissions, no one department would prioritize the feature addition very high.
AI could aggregate those complaints and help the product owner prioritize the development.
Conclusion
Current SaaS data analytics tools, while rich in features, often suffer from a complexity that hinders rather than helps.
The path to better data analytics lies in using AI to simplify these tools, making them more accessible and remove more barriers to doing good data work.
By allowing users to interact with these tools in a more intuitive way and by suggesting the most relevant analytics processes, AI can democratize data analysis, enabling a wider range of users to make data-driven decisions.
The integration of AI into data analytics tools will redefine our approach to data analysis.
The potential to transform these tools from complex, underutilized applications into essential, user-friendly platforms is enormous.
The question is no longer if this shift will happen, but when—and how quickly we can adapt to make the most of the future.